
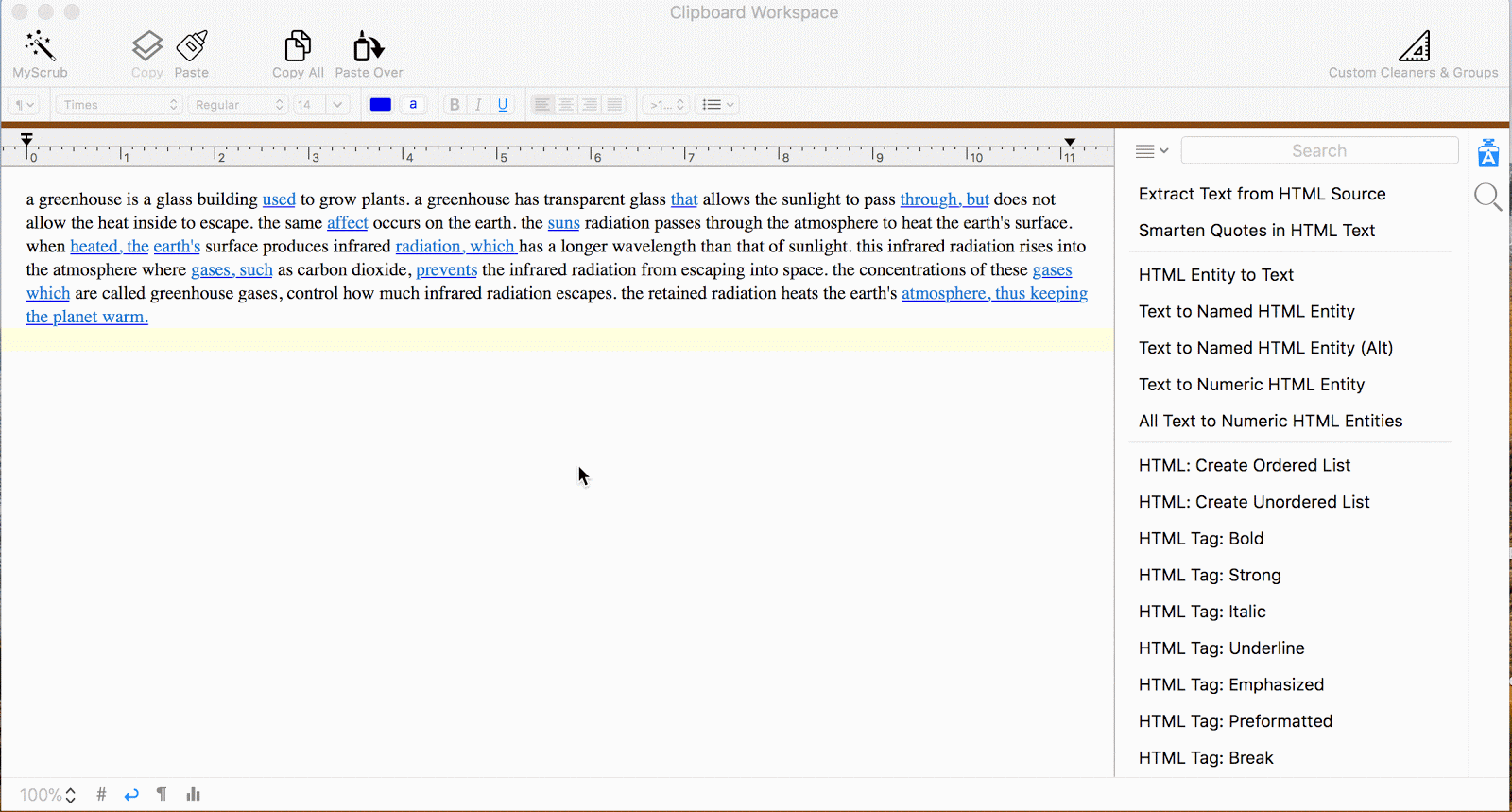
- #Nltk text cleaner how to#
- #Nltk text cleaner install#
- #Nltk text cleaner full#
- #Nltk text cleaner download#
- #Nltk text cleaner windows#
These are just a few of the many powerful commands available to process text in R. Here we will get back a character vector of length equal to the number of matches we found, containing the matches themselves. Note here that we used out first real regex - + which translates to "match any substring that is one or more contiguous numbers". Another thing I do all the time is extract all numbers (for example) from a string using the str_extract_all() function: str_extract_all(my_string,"+") If the function does not find anything to replace, it just returns the input unaltered. Note that the first argument is the object where we want to replace characters, the second is the thing we want to replace, and the third is what we want to replace it with. We can do this with the str_replace_all() function, which is detailed below: str_replace_all(my_string, "e","_")

The first replaces all instances of some character(s) with another character. There are two other very useful functions that I use quite frequently. Lets look at an example: grepl("\\?",my_string_vector) This function takes any number of strings as input and returns a logical vector of equal length with TRUE entries where a match was found, and FALSE entries where one was not. my_string differently than those without header tags, so using a conditional statement with a logical grep, grepl(), may be very useful to us. I also tend to use one of these programs to prototype any complex RegEx I want to use in production code. Whichever program you choose, I would suggest just messing around and reading random articles on the internet for a few hours before you get started using Regular Expressions in R. This program includes support for Perl style Regular Expressions which are quite common and are used by some R packages.
#Nltk text cleaner windows#
I personally prefer the RegExRx app, which should work on OSX and Windows and is available either as a shareware version or as a paid app on the Apple App Store. One simple way to do this is to use an online app with a graphical interface that highlights matches, such as the one provided here. If you want to get started using regular expressions, you can check out the tutorials posted above, but I have also found it very helpful to just start trying out examples and seeing how they work. What is important to understand is that they can be far more powerful than simple string matching. You can start by checking out this link to an overview of regular expressions, and then take a look at this primer on using regular expressions in R. If you want build your competency with text analysis in R, they are definitely a necessary tool. They are foundational to lots of different text processing tasks where we want to count types of terms (for example), or identify things like email addresses in documents. Regular expressions are a way of specifying rules that describe a class of strings (for example - every word that starts with the letter "a") that are more succinct and general than simply generating a dictionary and checking against every possible value that meets some rule.
#Nltk text cleaner download#
You can check out examples here, but download it from the first link above. MALLET does a whole bunch of useful statistical analysis of text, including an extremely fast implementation of LDA.They are much faster than the implementation in the OpenNLP R package. The Stanford CoreNLP libraries do a whole bunch of awesome things including tokenization and part-of-speech tagging.Here are links to my two favorite libraries:
#Nltk text cleaner how to#
I have also had success linking a number of text processing libraries written in other languages up to R (although covering how to do this is beyond the scope of this tutorial).
#Nltk text cleaner install#
I primarily make use of the stringr package for the following tutorial, so you will want to install it: install.packages("stringr", dependencies = TRUE) Furthermore, there is a lot of very active development going on in the R text analysis community right now (see especially the quanteda package). Yet there are good reasons to want to use R for text processing, namely that we can do it, and that we can fit it in with the rest of our analyses. Basic shell scripting can also be many orders of magnitude faster for processing extremely large text corpora - for a classic reference see Unix for Poets.
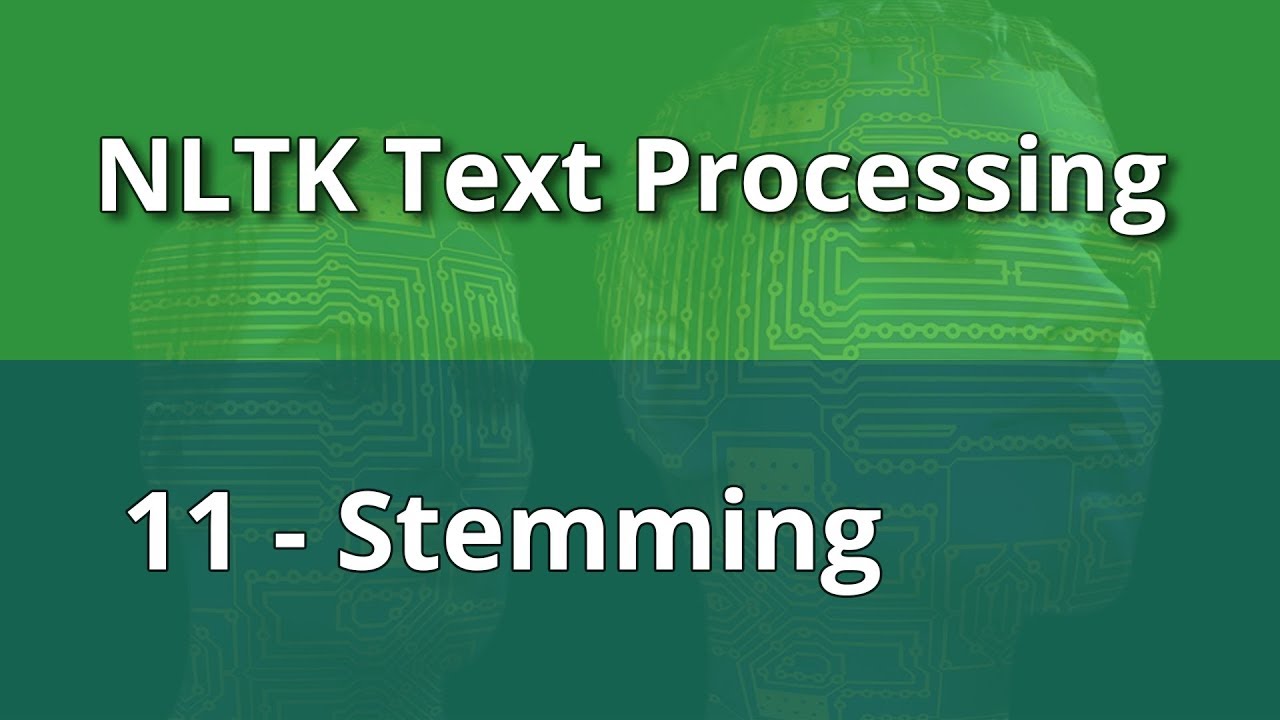
#Nltk text cleaner full#
Python is the de-facto programming language for processing text, with a lot of built-in functionality that makes it easy to use, and pretty fast, as well as a number of very mature and full featured packages such as NLTK and textblob. R is not the only way to process text, nor is it always the best way. This tutorial goes over some basic concepts and commands for text processing in R.
